Large language models are enabling private equity and executive search firms to leverage the power of their data like never before. In this ExitUp exclusive, Josh Gardner, chief technology officer at AiFlow, unpacks how this cutting-edge technology is driving returns and revenue across the PE and the human capital sectors.
As we kick off the new year, the AI revolution is well underway. ChatGPT now has 100 million weekly users and it only took 60 days to get there. Compare this to four and a half years for Facebook and 18 years for Netflix to reach the same milestone. For a technology that is so novel, its rapid adoption is nothing short of revolutionary.
Large language models perform a variety of natural language processing tasks. For private equity firms, LLMs are already simplifying tasks like due diligence and contract writing, and unleashing a torrent of new data on companies, contacts, and LPs. Deal sourcing, portfolio operations, and even investor relations are being revolutionized in unexpected ways. According to experts across the sector, the impact of generative AI on PE will be unprecedented. It is a game-changing, transformative technology that will give early adopters a vital competitive edge.
As we navigate this complex terrain, one standout player commands attention – AiFlow. The AI-native CRM company has been building tailored solutions that unleash the power of human capital for PE and executive search by using AI in novel ways. We have reason to believe that their technology will completely change the way that search and PE operate.
Ride the Wave, or Drown
AiFlow co-founders Nick Manske and Josh Gardner envision AiFlow as the catalyst that unlocks the full power of private equity and executive search firms’ networks. In a landscape where information sourcing and analysis are paramount, AiFlow enables organizations to find the right people for each use case in seconds, driving value from deal sourcing to deal closing to portfolio operations.
Understanding how this technology will change both the world and the way business is done necessitates understanding the technology itself.
This week, we sat down with AiFlow’s tech chief, Josh Gardner, to gain a better understanding of the overarching applications – and implications – of this new technology for high touch professions like private equity and executive search. AiFlow is transforming how LLMs are servicing the unique needs of PE firms and human capital providers, and we wanted to find out how.
If one thing became clear from our discussion, it is this: a large language model tsunami is on a collision course with private equity and executive search. Some firms are going to ride the wave while others will drown. Two camps of winners and losers will form, according to AiFlow, separated by those who understand and leverage the vast depths of this technology, and those who ignore it.
Josh, it is no secret that Silicon Valley has been investing heavily in LLMs. What do the numbers look like?
OpenAI has received $14 billion in funding from various venture capitalists, and large organizations such as Microsoft are banking their futures on the technology. Funding for their largest competitor, Anthropic, pales in comparison to this number. While OpenAI’s lead in fundraising is massive compared to any other single company, there are many LLM companies that VCs are pouring billions of dollars into collectively. With more companies cropping up every month, the LLM market is still anyone’s game. Investors are betting that there are going to be multiple winners.
“With more companies cropping up every month, the LLM market is still anyone’s game. Investors are betting that there are going to be multiple winners.”
What use cases are you already seeing for this technology in the PE and human capital spaces?
The use cases for large language model technology within private equity and human capital are pretty much boundless. These models are already being used to write job descriptions, perform due diligence, draft contracts, and write investment memos. The common thread here is using these models as writers, which makes sense because that’s what LLMs are exceptionally good at. However, there are some much less obvious use cases that represent the next wave of LLM adoption in the business world – mostly surrounding unlocking the potential of your existing data.
Is there a way to optimize LLMs to better service these sectors?
Resoundingly, yes! By fine tuning an LLM you can modify it to write in a specific style based on the role of the person using the program or the specific use case. I’ll give you an example from the talent space: recent studies have shown that the job descriptions written by Chat GPT get relatively low conversion rates, about five to 10 percent. But job descriptions written by the top billers in the recruiting world can often get conversion rates closer to 50%. This is where fine tuning comes in. If you take the job descriptions written by top recruiters, and feed them into ChatGPT, suddenly you can get your own custom version of ChatGPT that can write job descriptions that will get conversion rates that are also around 50%. This type of fine tuning can be applied to a ton of use cases. Fine tuning allows you to really harness the full power of LLMs based on your needs.
“LLMs are already being used to write job descriptions, perform due diligence, draft contracts, and write investment memos. But there are some much less obvious use cases that represent the next wave of LLM adoption in the business world.”
Where does LLM adoption in these verticals go from here? What does it look like?
There are two key technologies that must be understood to see how LLMs will be leveraged as the influx of data hits. The first one is tagging. LLMs are exceptionally good at tagging unstructured data, like resumes, contracts, websites, and customer reviews. Tagging occurs when an LLM takes unstructured data and labels it in ways that are well-formatted and easy to analyze quickly. Say you’re looking at resumes; what industries have people worked in? Even if industries aren’t mentioned directly in the resumes, LLMs can infer them based on their knowledge about companies. Remember, LLMs have read essentially the entire Internet. Continuing with the example, once the LLM tags resumes by industry, suddenly you can filter your resume database by industry. At a high level, if you show LLMs text data, they can label your data at a superhuman level – they know more than any one human being ever could and an work much faster and cheaper.
You mentioned a second technology?
In the process of creating LLMs, scientists had to create a related technology called an embedding. Embeddings take words and phrases and represent them in a conceptual way that the computer can reason about. Let’s say a user types “kitten” into a search bar. Using embeddings, the computer knows that a kitten is more like a cat than a dog, more like a dog than a wolf, and not even vaguely related to an apple. When you typically search things in various databases, say your CRM or LinkedIn, you often need to fiddle with keywords. By leveraging embeddings complex search processes can be simplified; you can type in a simple word and get everything conceptually related to the search. When you type “kitten” you’ll get cats too even if the word kitten is nowhere to be found. Combining this “conceptual search” with all the new data LLMs unlock and you get search quality that was completely impossible just one year ago.
“Firms that leverage their networks more effectively get better deals and more of them.”
How does AI flow leverage the synergy of these two technologies?
We have a client that is a software-focused growth equity firm with $10 billion in AUM. Over time, the firm has scaled from one to roughly 100 people. This scaling process has created data bloat; their CRM is filled with extremely outdated data and even data that is fresh is very poorly tagged. We’ve made it very easy for the firm to quickly run updates on their data and we helped them tag all of the data in their CRM so their search processes are more effective. We went through our client’s entire database to see if they were missing any information, and not only updated what they had in place but supplemented what they didn’t have. By tagging these fresh data sources into the client’s CRM they are now able to search their network in ways they couldn’t have even imagined before. This allowed them ultimately to move forward in the deal process more efficiently.
What benefits have your clients seen from their implementation of AiFlow?
By better leveraging their network they’ve been able to increase their assets under management and their IR team has been able to make more intros to potential LPs. The portfolio ops team has seen a significant increase in the number of customer intros and advisor intros. At the same time, the talent professionals on the portfolio ops team have seen a rapid decrease in the time to fill critical roles as it’s been much easier to leverage their network for referrals. All this together means higher deal flow, higher quality deals, and faster closing times.
Article By
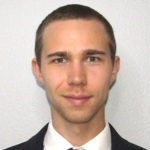
Caleb Edmundson
Caleb A. Edmundson is Editor-in-Chief of ExitUp, the investment blog from Hunt Scanlon Ventures designed for professionals across the human capital M&A sector. Caleb serves as an Associate for Hunt Scanlon Ventures, providing robust industry research to support the firm’s investment group. Connect with Caleb.